|
ACCESS THE FULL ARTICLE
No SPIE Account? Create one
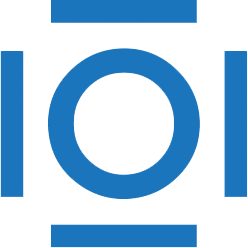
CITATIONS
Cited by 3 scholarly publications.
Fuzzy logic
Neural networks
Evolutionary algorithms
Detection and tracking algorithms
Neurons
Pattern recognition
Image classification