|
ACCESS THE FULL ARTICLE
No SPIE Account? Create one
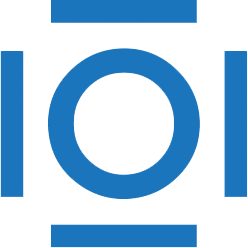
CITATIONS
Cited by 6 scholarly publications.
Automatic target recognition
Synthetic aperture radar
Target recognition
Image classification
Neural networks
Pattern recognition
Network architectures