|
ACCESS THE FULL ARTICLE
No SPIE Account? Create one
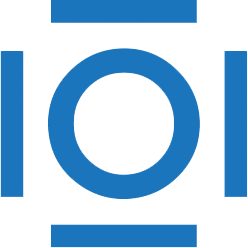
CITATIONS
Cited by 1 scholarly publication.
Image restoration
Autoregressive models
Image segmentation
Point spread functions
Satellites
Algorithm development
Earth observing sensors