|
ACCESS THE FULL ARTICLE
No SPIE Account? Create one
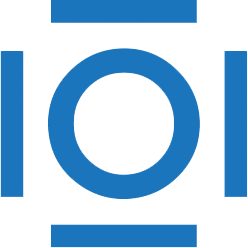
CITATIONS
Cited by 2 scholarly publications.
Databases
Dimension reduction
Principal component analysis
Hyperspectral imaging
Image analysis
Image processing algorithms and systems
Matrices