|
ACCESS THE FULL ARTICLE
No SPIE Account? Create one
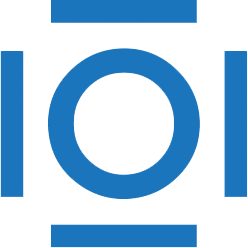
CITATIONS
Cited by 11 scholarly publications.
Prostate
Signal to noise ratio
Feature extraction
Tissues
Image classification
Image segmentation
Cancer