|
ACCESS THE FULL ARTICLE
No SPIE Account? Create one
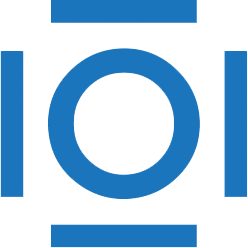
CITATIONS
Cited by 2 scholarly publications.
Image segmentation
Field programmable gate arrays
Image processing algorithms and systems
Image classification
Algorithm development
Image quality
Neural networks