|
ACCESS THE FULL ARTICLE
No SPIE Account? Create one
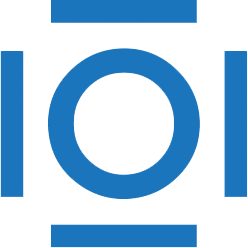
CITATIONS
Cited by 2 scholarly publications.
Image segmentation
Multispectral imaging
Distance measurement
Feature selection
Cervical cancer
Machine learning
Computer aided diagnosis and therapy