|
ACCESS THE FULL ARTICLE
No SPIE Account? Create one
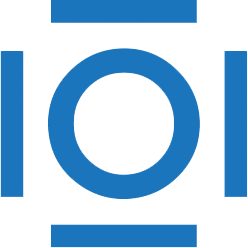
CITATIONS
Cited by 1 scholarly publication.
3D acquisition
Atmospheric sensing
Data modeling
Hyperspectral imaging
3D modeling
Environmental sensing
Error analysis