|
ACCESS THE FULL ARTICLE
No SPIE Account? Create one
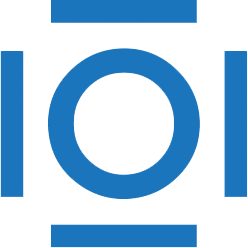
CITATIONS
Cited by 7 scholarly publications.
Gait analysis
Data fusion
Biometrics
Motion models
Databases
Feature extraction
Motion estimation