|
ACCESS THE FULL ARTICLE
No SPIE Account? Create one
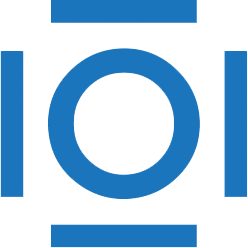
CITATIONS
Cited by 2 scholarly publications.
Particles
Detection and tracking algorithms
Signal to noise ratio
Sensors
Algorithm development
Monte Carlo methods
Surveillance