|
ACCESS THE FULL ARTICLE
No SPIE Account? Create one
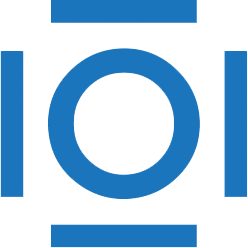
CITATIONS
Cited by 2 scholarly publications.
Object recognition
Binary data
Field programmable gate arrays
Video
Databases
Feature extraction
Pattern recognition