|
ACCESS THE FULL ARTICLE
No SPIE Account? Create one
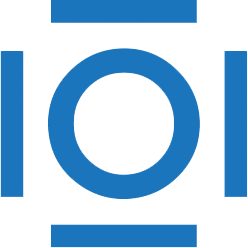
CITATIONS
Cited by 2 scholarly publications.
Neural networks
Video
Video processing
Data processing
Evolutionary algorithms
Neurons
Detection and tracking algorithms