|
ACCESS THE FULL ARTICLE
No SPIE Account? Create one
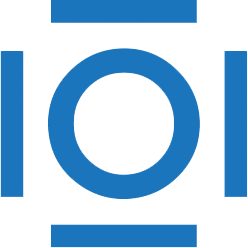
CITATIONS
Cited by 2 scholarly publications.
Biomedical optics
Magnetic resonance imaging
Visualization
Brain mapping
Data analysis
Data modeling
Associative arrays