|
ACCESS THE FULL ARTICLE
No SPIE Account? Create one
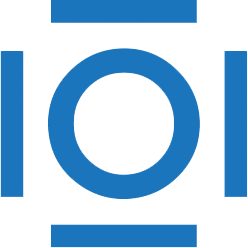
CITATIONS
Cited by 1 scholarly publication.
Magnetoencephalography
Data modeling
Functional magnetic resonance imaging
Expectation maximization algorithms
Error analysis
Neuroimaging
Electroencephalography