|
ACCESS THE FULL ARTICLE
No SPIE Account? Create one
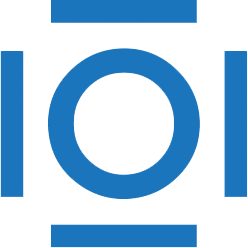
CITATIONS
Cited by 9 scholarly publications.
Independent component analysis
Functional magnetic resonance imaging
Data modeling
Brain
Brain imaging
Data fusion
Neuroimaging