|
ACCESS THE FULL ARTICLE
No SPIE Account? Create one
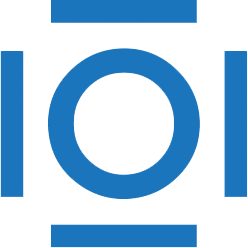
CITATIONS
Cited by 2 scholarly publications.
Stars
Astronomy
Feature selection
Feature extraction
Galactic astronomy
Observatories
Radio optics