|
ACCESS THE FULL ARTICLE
No SPIE Account? Create one
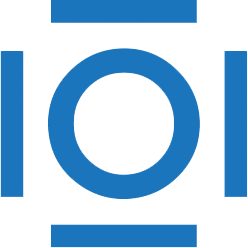
CITATIONS
Cited by 27 scholarly publications and 1 patent.
Computed tomography
Data modeling
Tomography
Bismuth
Radon transform
Reconstruction algorithms
CT reconstruction