|
ACCESS THE FULL ARTICLE
No SPIE Account? Create one
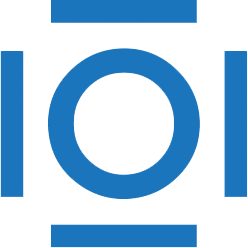
CITATIONS
Cited by 5 scholarly publications.
Image segmentation
Prostate
Statistical modeling
Magnetic resonance imaging
3D modeling
3D image processing
Principal component analysis