|
ACCESS THE FULL ARTICLE
No SPIE Account? Create one
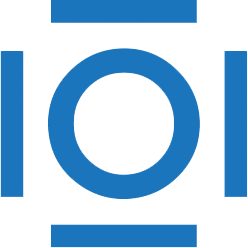
CITATIONS
Cited by 1 patent.
Detection and tracking algorithms
Wavelets
Environmental sensing
Sensor networks
Sun
Optical sensors
Discrete wavelet transforms