|
ACCESS THE FULL ARTICLE
No SPIE Account? Create one
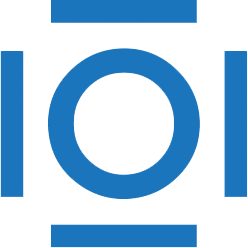
CITATIONS
Cited by 1 scholarly publication.
Data modeling
Brain
Feature extraction
Functional magnetic resonance imaging
Error analysis
Magnetorheological finishing
Statistical analysis