|
ACCESS THE FULL ARTICLE
No SPIE Account? Create one
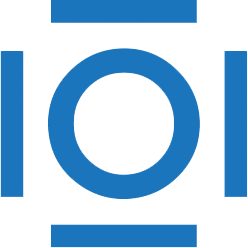
CITATIONS
Cited by 7 scholarly publications.
Prisms
Magnetic resonance imaging
Coherence (optics)
Temporal resolution
Image quality
Data acquisition
Computed tomography