|
ACCESS THE FULL ARTICLE
No SPIE Account? Create one
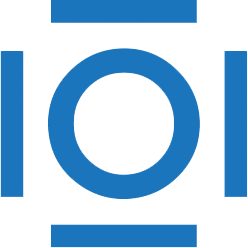
CITATIONS
Cited by 5 scholarly publications.
Tissues
Software development
Optical coherence tomography
Image classification
Image processing
Image analysis
Feature extraction