|
ACCESS THE FULL ARTICLE
No SPIE Account? Create one
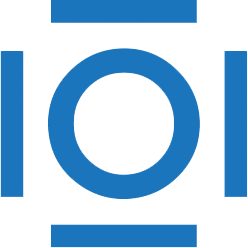
CITATIONS
Cited by 5 scholarly publications.
Land mines
Data modeling
General packet radio service
Statistical modeling
Detection and tracking algorithms
Ground penetrating radar
Expectation maximization algorithms