|
ACCESS THE FULL ARTICLE
No SPIE Account? Create one
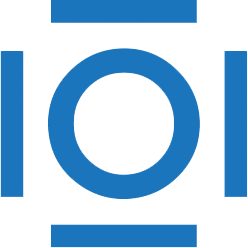
CITATIONS
Cited by 1 scholarly publication.
Hyperspectral imaging
Image filtering
Joint transforms
Fourier transforms
Optical filters
Detection and tracking algorithms
Image classification