|
ACCESS THE FULL ARTICLE
No SPIE Account? Create one
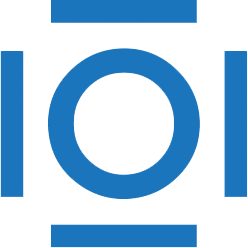
CITATIONS
Cited by 4 scholarly publications.
Data fusion
Data modeling
Sensors
Systems modeling
Intelligence systems
Data processing
Performance modeling