|
ACCESS THE FULL ARTICLE
No SPIE Account? Create one
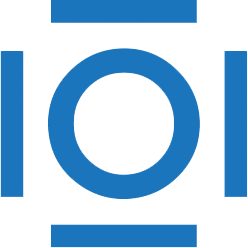
CITATIONS
Cited by 2 scholarly publications.
Image segmentation
Electrochemical etching
Control systems
Performance modeling
Visualization
Electrical engineering
Statistical modeling