|
ACCESS THE FULL ARTICLE
No SPIE Account? Create one
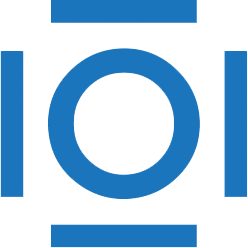
CITATIONS
Cited by 2 scholarly publications.
Motion models
Principal component analysis
Motion estimation
Magnetic resonance imaging
Nuclear medicine
Expectation maximization algorithms
Chest