|
ACCESS THE FULL ARTICLE
No SPIE Account? Create one
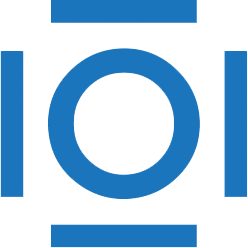
CITATIONS
Cited by 3 scholarly publications.
Computing systems
Mammography
Solid modeling
Visual process modeling
Feature extraction
Radiology
Systems modeling