|
ACCESS THE FULL ARTICLE
No SPIE Account? Create one
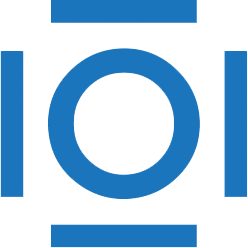
CITATIONS
Cited by 3 scholarly publications.
Pulmonary function tests
Stanford Linear Collider
Remote sensing
Detection and tracking algorithms
Feature selection
Reflectivity
Algorithm development