|
ACCESS THE FULL ARTICLE
No SPIE Account? Create one
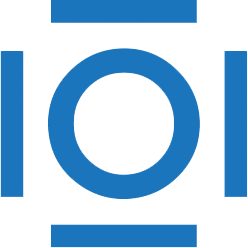
CITATIONS
Cited by 4 scholarly publications.
Databases
Image quality
Distortion
Image compression
Evolutionary algorithms
Neural networks
Statistical analysis