|
ACCESS THE FULL ARTICLE
No SPIE Account? Create one
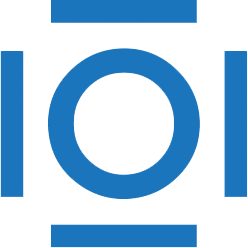
CITATIONS
Cited by 1 scholarly publication.
Data modeling
Mining
Databases
Fourier transforms
Performance modeling
Computer simulations
Expectation maximization algorithms