|
ACCESS THE FULL ARTICLE
No SPIE Account? Create one
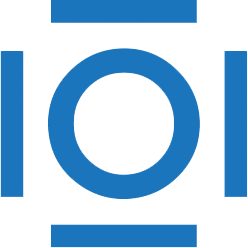
CITATIONS
Cited by 1 scholarly publication.
Evolutionary algorithms
Binary data
Image processing
Matrices
Bismuth
Neurons
Computer simulations