|
ACCESS THE FULL ARTICLE
No SPIE Account? Create one
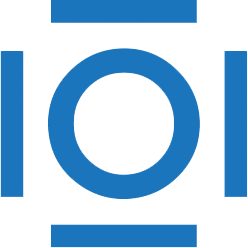
CITATIONS
Cited by 2 scholarly publications.
Gadolinium
Neural networks
Aluminum
Calibration
Polymethylmethacrylate
Chest
Error analysis