|
ACCESS THE FULL ARTICLE
No SPIE Account? Create one
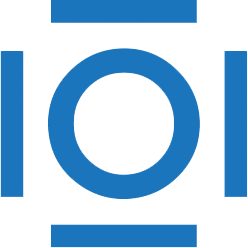
CITATIONS
Cited by 3 scholarly publications.
Image segmentation
Computed tomography
Natural surfaces
Picosecond phenomena
Tissues
Cancer
Feature extraction