|
ACCESS THE FULL ARTICLE
No SPIE Account? Create one
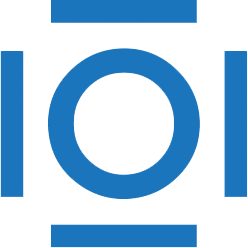
CITATIONS
Cited by 39 scholarly publications and 2 patents.
Convolution
Digital breast tomosynthesis
Neural networks
Reconstruction algorithms
CAD systems
Computer architecture
Computer aided diagnosis and therapy