|
ACCESS THE FULL ARTICLE
No SPIE Account? Create one
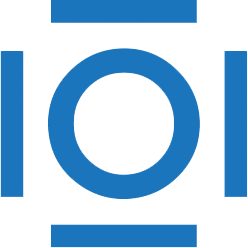
CITATIONS
Cited by 1 scholarly publication.
Wavelets
Detection and tracking algorithms
Gesture recognition
Fast wavelet transforms
Data modeling
Feature selection
Statistical modeling