Photonic lanterns (PLs) allow the decomposition of highly multimodal light into a simplified modal basis such as single-moded and/or few-moded. They are increasingly finding uses in astronomy, optics, and telecommunications. Calculating propagation through a PL using traditional algorithms takes ∼1 h per simulation on a modern CPU. We demonstrate that neural networks can bridge the disparate opto-electronic systems and, when trained, can achieve a speedup of over five orders of magnitude. We show that this approach can be used to model PLs with manufacturing defects and can be successfully generalized to polychromatic data. We demonstrate two uses of these neural network models: propagating seeing through the PL and performing global optimization for purposes such as PL funnels and PL nullers. |
ACCESS THE FULL ARTICLE
No SPIE Account? Create one
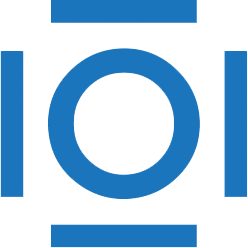
CITATIONS
Cited by 1 scholarly publication.
Waveguides
Data modeling
Wavefronts
Lithium
Neural networks
Zernike polynomials
Broadband telecommunications