|
ACCESS THE FULL ARTICLE
No SPIE Account? Create one
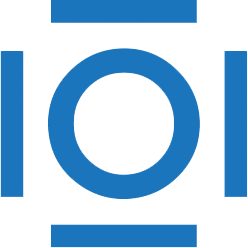
CITATIONS
Cited by 23 scholarly publications.
Hyperspectral imaging
Image segmentation
Image classification
Spatial resolution
Sensors
Artificial neural networks
Associative arrays