|
ACCESS THE FULL ARTICLE
No SPIE Account? Create one
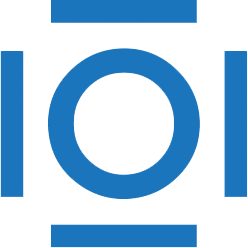
CITATIONS
Cited by 4 scholarly publications.
Hyperspectral imaging
Image classification
Remote sensing
Lutetium
Spatial resolution
Communication engineering
Machine learning