|
ACCESS THE FULL ARTICLE
No SPIE Account? Create one
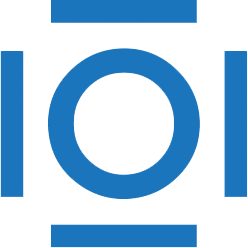
CITATIONS
Cited by 20 scholarly publications.
Convolution
Image classification
Hyperspectral imaging
3D modeling
Feature extraction
Data modeling
3D image processing