|
ACCESS THE FULL ARTICLE
No SPIE Account? Create one
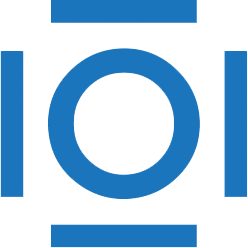
CITATIONS
Cited by 7 scholarly publications.
Feature extraction
Convolution
Convolutional neural networks
Absorption
3D modeling
Scene classification
Image classification