|
ACCESS THE FULL ARTICLE
No SPIE Account? Create one
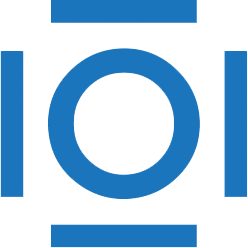
CITATIONS
Cited by 26 scholarly publications.
Data fusion
Remote sensing
Roads
Visualization
Data modeling
Image classification
Image fusion