In many areas of the globe, the installation of artificial drains on naturally poorly drained soils is a necessary part of farm management. Identifying the location of artificially drained areas is an important step in achieving environmentally sustainable agricultural production. However, in many regions, data on the presence or the distribution of artificial drainage systems are rare. We outline an approach to identify artificially drained soils using Earth observation (EO) satellite imagery and digital elevation data. The method exploits the contrasting phenology of grass during a peak growth stage to identify artificially drained and undrained soils. Two machine-learning techniques, support vector machine and random forest, were tested. Classification accuracy up to 91% was achieved using photointerpreted accuracy points using higher resolution satellite imagery. Additional investigations would be required to establish whether the drained conditions identified were a result of artificial drainage or from naturally well-drained soils occurring within larger soil units. Herein, the Republic of Ireland is used as a test case. Based on our findings, the area of artificially drained grassland within the study area could be revised upward, with 44% (or ∼345 , 000 ha) of pasture currently classed as “poorly drained” identified as “artificially drained.” At one location, a change in the modeled drainage condition at field level was demonstrated following drain installation. The presented method demonstrates the ability of EO satellites to quickly and accurately map field drainage status at farm management scales over a wide area. This has the potential to improve management decisions at local scales, but also has implications in terms of national policy development and regulation in areas such as water quality and climate change mitigation. |
ACCESS THE FULL ARTICLE
No SPIE Account? Create one
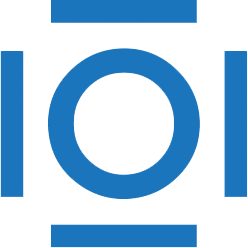
CITATIONS
Cited by 3 scholarly publications.
Earth observing sensors
Landsat
Soil science
Data modeling
Machine learning
Reflectivity
Satellites