Net surface radiation defines the availability of radiation energy on and near the surface to drive many physical and physiological processes such as latent heat, sensible heat fluxes, and evapotranspiration. One of the prime challenges of modeling radiation budget is estimation of net longwave radiation. Incoming or downwelling longwave radiation (LWin) flux is one of the two key components of net longwave radiation. Its estimation in cloudy conditions has always been a challenge due to lack of instrumentation and regular measurements at different spatial scales. In this study, two artificial neural network (ANN) multi-layer perceptron (MLP) models were developed for LWin flux estimation under cloudy-sky during daytime and nighttime using half-hourly flux measurements over different agro-climatic settings and several atmospheric parameters from measurements, satellite-based observations, and model outputs. A comparative evaluation was made between existing or newly developed multivariate linear regression (MVR) models and ANN-based models. The latter set of models were found to be superior to the best MVR model during both daytime and nighttime. The ANN models were found to have consistent performance across different sites and cloud types except less accuracy in sub-humid or humid climate and in deep convection cloud. The ANN models showed overall accuracies of 2.7% and 3.3% of measured mean and R2 of 0.86 and 0.85 for daytime and nighttime, respectively, when compared with independent data of in-situ measurements. |
ACCESS THE FULL ARTICLE
No SPIE Account? Create one
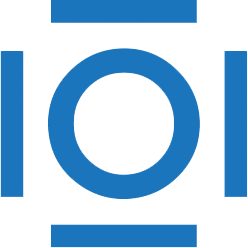
CITATIONS
Cited by 4 scholarly publications.
Clouds
Atmospheric modeling
Neural networks
Performance modeling
Commercial off the shelf technology
Solar radiation models
Data modeling