Sparsity and low rankness are essential properties of real-world data. The sparsity attribute mentions that the data contain very few non-zero elements. On the other hand, the low-rank property characterizes redundancy in the data. The concept of sparsity and low rankness is central to numerous signal processing tasks such as signal inversion, data compression, and noise removal. With the advent of multi-sensor and multi-dimensional data, we frequently encounter multi-way data or tensors with sparse and low-rank structure. Prevalent vector or matrix-based sparsity estimation criterias cannot conveniently characterize the sparsity structure of multi-way data. Hence, we require efficient criteria to quantify the sparsity structure of multi-way data. Sparsity plays a crucial aspect in hyperspectral image processing tasks such as unmixing and denoising. Hyperspectral images contain an inherent multi-way structure; we perform multi-way sparsity estimation in hyperspectral image processing. We introduce an efficient criterion to estimate multi-way sparsity, facilitating signal inversion, and noise removal tasks. The proposed sparsity criteria combine the sparsity of the core tensor obtained by Tucker tensor decomposition, the low rankness of the tensor, and the approximated rank. Since the core tensor obtained by tucker decomposition is highly sparse, the core tensor sparsity reflects the sparsity of the whole tensor indirectly. On the other hand, the nuclear norm and Stein’s unbiased risk estimate quantifies the low rankness of the tensor. Unlike other approaches, our proposed measure takes both low rankness and sparsity attribute into account. In addition, our proposed sparsity measure also satisfies some of the desirable properties of ideal sparsity measures. We demonstrate the efficacy of our proposed sparsity quantification measure in applications such as hyperspectral image denoising, hyperspectral unmixing tasks as demonstrated in the real image experiments. |
ACCESS THE FULL ARTICLE
No SPIE Account? Create one
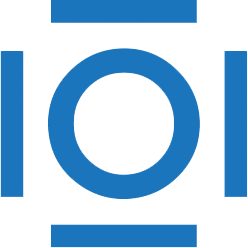
CITATIONS
Cited by 4 scholarly publications.
Hyperspectral imaging
Signal to noise ratio
Denoising
Image analysis
Matrices
Associative arrays
Image denoising