Hyperspectral image (HSI) classification has many applications in different diverse research fields. We propose a method for HSI classification using principal component analysis (PCA), 2D spatial convolution, and support vector machine (SVM). Our method takes advantage of correlation in both spatial and spectral domains in an HSI data cube at the same time. We use PCA to reduce the dimensionality of an HSI data cube. We then perform spatial convolution to the dimension-reduced data cube once and then to the convolved data cube for the second time. As a result, we have generated two convolved PCA output data cubes in a multiresolution way. We feed the two convolved data cubes to SVM to classify each pixel to one of the known classes. Experiments on three widely used hyperspectral data cubes (i.e., Indian Pines, Pavia University, and Salinas) demonstrate that our method can improve the classification accuracy significantly when compared to a few existing methods. Our method is relatively fast in terms of central processing unit computational time as well. |
ACCESS THE FULL ARTICLE
No SPIE Account? Create one
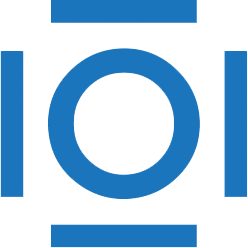
CITATIONS
Cited by 2 scholarly publications.
Principal component analysis
Convolution
Image classification
Hyperspectral imaging
Composites
Remote sensing
MATLAB