Deep convolutional neural network semantic segmentation has played a significant role in remote sensing applications due to its capability of end-to-end training and automatic high-level features extraction. Highly accurate predictions have been recorded from different network architectures that are designed using the convolutional layers. However, the accuracy is mainly achieved at a very high cost of computation, which renders the network infeasible for real-time application on resource-constrained devices. Therefore, there is a need to establish a trade-off between accuracy and model efficiency. Our paper proposes a lightweight, highly accurate, memory-efficient segmentation network capable of deployment on resource-constrained devices. Our proposed network of 1.09 million trainable parameters attains an appreciable accuracy of 89.41% and 88.78% on the Vaihingen and Potsdam respective dataset of the ISPRS 2D Semantic Labeling Challenge. The result from the speed and the memory efficiency experiment shows that our proposed network is suitable for real-time remote sensing applications. |
ACCESS THE FULL ARTICLE
No SPIE Account? Create one
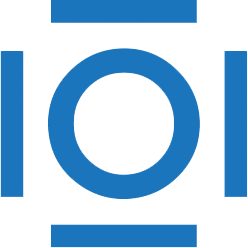
CITATIONS
Cited by 5 scholarly publications.
Image segmentation
Remote sensing
Convolution
Network architectures
Data modeling
Feature extraction
Performance modeling