The scale-invariant feature transform (SIFT) algorithm is widely used in remote sensing optical image matching because of its robustness and universality. However, owing to the large amount of data and complex and nonlinear change spectral information of multisource and multiscale optical remote sensing images, the matching performance of classic SIFT and related improved algorithms is not ideal. To mitigate this, we propose an SIFT matching algorithm for remote sensing optical images based on entropy classification optimization. After using the classic SIFT to extract features based on the entropy classification standard, the features are classified to reduce the number of invalid features in the matching process and high-precision transformation parameters. Then, the projection model is used to detect stable features to obtain correctly matched pairs, which addresses the problem of minimal matched pairs due to complex and nonlinear changes. We compared it with commonly used algorithms, such as the SIFT, uniformly robust SIFT, and scale normalization and size classification algorithms. The proposed algorithm was successfully applied to multisource and multiscale remote sensing images. Experimental results show that the proposed algorithm performs better than the comparison algorithms in terms of precision, distribution quality, and in matching highly differing images. |
ACCESS THE FULL ARTICLE
No SPIE Account? Create one
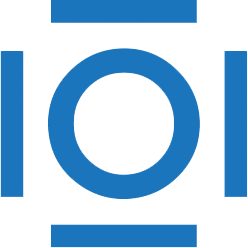
CITATIONS
Cited by 2 scholarly publications.
Remote sensing
Image classification
Spatial resolution
Image processing
Image sensors
Image fusion
Image quality