|
ACCESS THE FULL ARTICLE
No SPIE Account? Create one
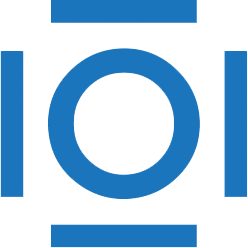
CITATIONS
Cited by 13 scholarly publications.
Radar
Machine learning
Agriculture
Remote sensing
Sensors
Polarization
Backscatter