1.IntroductionCerebral blood flow (CBF) performs an essential role in satisfying the metabolic needs of the brain by transporting glucose and oxygen to the brain tissue and clearing metabolic waste, such as , from it via a dense microvascular network. The crucial mechanism that accounts for continuously adjusting CBF to the local metabolic needs is defined as neurovascular coupling (NVC),1,2 and its status correlates with brain cognitive function.3–5 The NVC also plays a vital role in interpreting brain functioning using imaging techniques, such as functional magnetic resonance imaging and positron emission tomography.6,7 The NVC relies on the coordination of multiple signaling pathways involving various cell types in the brain, such as neurons, astrocytes, pericytes, as well as vascular smooth muscle cells and endothelial cells.8,9 On the other hand, NVC can be impaired under various pathological conditions, such as Alzheimer’s disease,10–13 hypertension,14 atherosclerosis,15 and seizures.16 Therefore, the accurate mapping of CBF velocities from a dense microvascular network could be a key step toward a better understanding of NVC in both normal and pathological brain conditions. In addition, it could also contribute to the development of more accurate numerical models of oxygen delivery and consumption in realistic microvascular networks.17,18 A suitable method to achieve this task should be capable of (i) obtaining accurate cerebrovascular angiograms with microscopic resolution that will include a majority of or all arterioles, venules, and capillaries over a large field of view (FOV) and (ii) acquiring volumetric blood flow velocity data from the majority of the microvascular segments over the same FOV. Several imaging modalities, such as multiphoton microscopy (MPM)19–21 [e.g., two-photon microscopy (2PM) and three-photon microscopy (3PM)], optical coherence tomography (OCT),22–25 photoacoustic microscopy (PAM),26–29 and 3D ultrasound localization microscopy (ULM)30–33 are capable of acquiring both microvascular angiograms and blood flow in most cortical microvascular segments. Among these imaging methods, MPM is unique in its ability to provide more accurate morphological parameterization of the microvascular networks in vivo. It is the preferred method to acquire accurate capillary morphology in vivo, across the mouse cortical layers (-thick) and over a large FOV (up to ). On the other hand, MPM, OCT, PAM, and ULM are all capable of acquiring large microvascular velocity maps in a short time, which is very important for in vivo imaging studies. MPM typically employs a line scan method to measure the blood flow velocity that is perpendicular to the optical axis direction for arteries, veins, and capillaries. For each vessel segment, the line scan is performed repeatedly at a 1 to 2 kHz rate along its longitudinal direction until it is sufficient to determine the flow velocity, and is a reasonable target for measurement temporal resolution,19 which renders it only suitable for retrieving cerebral flow velocity values from a limited number of vessel segments. Faster volumetric scanning MPM has been enabled by raster-scanning a Bessel beam34 and blood flow velocities can be rapidly acquired with this method over a limited FOV.35 Recently, an ultrafast free-space angular chirp enhanced delay scanning protocol has increased the temporal resolution for two-photon cortical blood flow imaging by orders of magnitude to 2D frame rate.36 PAM is a label-free method for wide-FOV (e.g., several ) and relatively deep (e.g., imaging depth) mapping of total hemoglobin concentration (), oxygen saturation of hemoglobin (), and blood flow speed by making use of the absorption contrast, weak acoustic scattering in soft tissue, and high optical resolution (lateral: 2 to and axial: ).37 Fast 3D volumetric imaging at is achievable with PAM over a 4 to FOV.26,28 ULM achieves super-resolution microvascular imaging by tracking microbubbles (diameter: 1 to ) administered into the bloodstream of the subjects and utilizing the super-resolution concept from optical microscopy. ULM is able to map the microvasculature from the entire mouse brain with data acquisition time of several tens of seconds, spatial resolution of , and sensitivity to flow velocities between 2 and .33 However, ULM requires the localization of individual microbubbles in the bloodstream. For imaging the small vessels, this is a time-consuming procedure and it may take tens of minutes to resolve the capillaries.32 OCT has been widely used for mapping absolute axial blood flow based on the Doppler effect that results from the motion of RBCs. The common Doppler OCT method usually acquires dense A-scans within a B-scan to resolve the phase change, and typical imaging time for such a B-scan is .22,23 Capillary blood flow velocities can also be obtained by analyzing the dynamic scattering component of the OCT signals using the power spectrum bandwidth of the autocorrelation function38 or laser speckle decorrelation time.39 Recently, dynamic light scattering optical coherence tomography (DLS-OCT)40–42 was proposed to measure absolute blood flow velocity and the RBC diffusion coefficient. DLS-OCT can provide volumetric imaging over a FOV of and imaging depth, with isotropic spatial resolution and with -long data acquisition time for a single volume. This is achieved by utilizing the DLS approach for estimating RBC velocities and diffusion coefficients and has proved to be less susceptible to noise and more sensitive to RBC motion than Doppler OCT,43 leading to reliable blood flow velocity measurements in the capillaries. OCT is also capable of obtaining the microvascular angiograms44 but, similar to PAM and ULM, with inferior quality to the MPM angiograms, which renders it less optimal for accurate image segmentation and quantitative analysis of microvascular morphology. While multiple imaging modalities may be used to measure the microvascular CBF velocities, OCT has been among the most common tools applied for this task and it is optically highly compatible with MPM. Therefore, we selected a combination of DLS-OCT and 2PM to acquire both the CBF velocities from a large number of microvascular segments and high-resolution microvascular angiograms, respectively. It is worth noting that the framework developed based on the combination of DLS-OCT and 2PM can be extended to accommodate other possible multimodal imaging strategies, such as OCT and 3PM, which has gained traction over the past several years for acquiring angiograms with an increased imaging depth compared to 2PM. However, it is very difficult to integrate OCT and MPM together for simultaneous multimodal imaging without sacrificing their optimal performance. For example, objective lenses with high numerical aperture (NA) () and properly filled back aperture by the laser beam are typically used in 2PM to achieve lateral resolution and sufficient fluorescence emission signal collection efficiency, whereas using the same objective lens with a filled back aperture in an OCT system will create a de facto optical coherence microscope with extremely small depth of focus, which will prevent efficient acquisition of volumetric cerebral angiograms and blood flow velocity in mouse brain. In addition, MPM and OCT require significantly different scanning protocols, which are difficult to achieve if the excitation beams are scanned simultaneously by the same scanning mirrors. Although various attempts could be made to overcome these problems, such as drastically underfilling of the objective back aperture with the OCT beam to increase the depth of focus of OCT or using a Bessel beam to extend the OCT imaging range in the axial direction when using high-NA objective lenses, they will significantly complicate the imaging setup and make it much less practical. On the other hand, utilizing separate scanning mirrors for 2PM and OCT removes the constraints on scanning protocols, but it introduces different optical distortions to two imaging modalities and, therefore, requires advanced 3D coregistration. In this work, we developed a computational framework to co-register large-scale microvascular blood flow velocity measurements from DLS-OCT with the high-resolution microvascular angiograms obtained using 2PM over the same FOV. We first performed a global 3D coregistration and a multilayer 2D coregistration to transform the 2PM angiogram onto the DLS-OCT angiogram. Then we segmented the original 2PM microvascular angiogram, obtained its mathematical graph representation, and transformed the vectorized angiogram onto the DLS-OCT image space based on the transformation matrices computed in the first step. Finally, the DLS-OCT flow velocity measurements were mapped onto the 2PM angiogram and mean microvascular segment velocities were extracted. The developed framework was implemented to process the datasets acquired from the three mice cortices. The distributions of mean CBF velocities in arterioles, venules, and capillaries as a function of vessel type and the branching order from precapillary arterioles and postcapillary venules have been retrieved. The framework proposed here will facilitate the numerical modeling of CBF and oxygen transport within cerebral microvascular networks and help us improve our understanding of the microvascular blood flow regulation in normal brain and in various brain conditions. 2.Methods2.1.Animal PreparationThree healthy, young-adult female C57BL/6 mice ( months old; The Jackson Laboratory, Maine, United States) were used in this study. A sealed 3-mm-diameter cranial window was installed over the whisker barrel cortex area (on the left hemisphere; posterior to bregma and lateral from the midline). Tracheotomy was performed to enable controlled ventilation and maintain proper physiological status in subjects by providing a mixture of medical air () and isoflurane (1.2% to 1.5%). A femoral artery catheter was installed to facilitate the administration of a contrast agent in 2PM imaging and to monitor physiological parameters, such as blood pressure, heart rate, and blood gases. Mice were kept under isoflurane anesthesia during the subsequent imaging sessions. 2.2.DLS-OCT ImagingDLS-OCT was performed using a spectral domain OCT setup42 (Thorlabs Inc., New Jersey, United States) [Fig. 1(a)]. The setup utilizes an extended broadband superluminescent diode (SLD) source (LS2000B, Thorlabs Inc., New Jersey, United States) that implements two matched-pair SLDs to offer bandwidth at center wavelength. A objective (MPlanApoNIR, , Mitutoyo, Japan) was used in the experiments. An InGaAs line scan camera with 1024 pixels was used to record the interference signal at 46,000 A-scans/s. The system can achieve spatial resolution in all three dimensions within the brain tissue and maximum imaging depth. OCT en face images of the pial surface were obtained for selecting a FOV (). In this study, we only imaged down to beneath the brain surface of each subject considering the confocal parameter of the system since it provided a sufficient number of arterioles, venules, and capillaries to demonstrate the proposed framework. We first performed OCT angiography and repeated B-scan acquisition protocol was employed. The final 3D angiogram was obtained with 10 times averaging, which took 88 s for data acquisition. For the blood flow speed measurements using DLS-OCT, an -mode data acquisition protocol with 100 A-scan repeats at each transverse location was employed and the data acquisition time for a (voxel size: ) volume was . Since the measurement of a component of flow velocity that is parallel with the optical axis (Vz) has the largest SNR of all DLS-OCT velocity estimates, we relied on it to calculate the microvascular flow velocity based on the angle between vessel direction and the optical axis of the system. Fig. 1The diagrams of the OCT and 2PM imaging setups. (a) Diagram of the spectral domain OCT system. SLDs, superluminescent diodes; FC, fiber coupler; CLs, collimating lenses; DC, dispersion compensation; RM, reference mirror; M, mirror; GM, galvo mirrors; SL, scan lens; TL, tube lens; DM, dichroic mirror; OL, objective lens; DG, diffraction grating; SOL, spectrometer objective lens; LCCD, line-scan CCD; DAQ, data acquisition card; and PC, computer. (b) Diagram of the 2PM system. M1 and M2: mirrors; EOM, electro-optic modulator; SH, shutter; GM, galvanometer mirrors; SL, scan lens; TL, tube lens; DM, dichroic mirror; OL, objective lens; FFs, fluorescence filters; PMTs, photomultiplier tubes; DAQ, data acquisition card; and PC, computer. ![]() 2.3.2PM AngiographyThe 2PM angiograms were acquired after DLS-OCT measurements using a custom-built 2PM setup45 [Fig. 1(b)]. A mode-locked laser (Insight DeepSee, pulse width, 80 MHz pulse repetition rate, Spectra-Physics, California, Unites States) tunable between 680 and 1300 nm was used for the 2P excitation. The output power of the laser was controlled by an electro-optic modulator (EOM) (Model 350-160, ConOptics Inc., Connecticut, United States). Beam scanning in the plane was achieved by a set of galvanometer mirrors (6215H, Cambridge Technology Inc., Massachusetts, United States) with laser beam relayed by a scan lens (, AC254-030-B, Thorlabs Inc., New Jersey, United States) and a tube lens (, Olympus, Japan) to the back aperture of the objective. A water immersion objective lens (XLUMPLFLN20XW, NA = 1.00, Olympus, Japan) was used to focus the beam on the sample. The far-red fluorescent dye Alexa Fluor 680 conjugated to 70 kDa dextran was used to label blood plasma as the contrast agent for 2PM (, 0.1 mL). The emission signal of Alexa Fluor 680 was detected using a photomultiplier tube (PMT) (H10770PA-50, Hamamatsu, Japan), a dichroic mirror (FF875-Di01-, Semrock, New York, United States), and two fluorescence filters (FF01-890/SP-50 and FF01-709/167-25, Semrock, New York, United States). In this study, 2PM survey images of the pial surface were first acquired and, with help of previously acquired OCT en face images, used to define an ROI () for 2PM that contained the ROI of DLS-OCT. The 2PM angiogram was subsequently acquired down to an imaging depth (voxel size: ). The use of animals in this study was approved by the Institutional Animal Care and Use Committee at Massachusetts General Hospital. Typical examples of the volumetric datasets acquired from the two imaging sessions are visualized in Figs. 2(a)–2(c). 2.4.Angiogram Segmentation and GraphingFor quantitative modeling and analysis of cerebral microvascular networks, graph-based representations of the structure are needed.46,47 The 2PM angiograms were analyzed using Amira software (Thermo Fisher Scientific, Massachusetts, United States) combined with custom software written in C++, running on a graphical processor unit equipped computer, in the following steps. (i) Adaptive contrast enhancement. For each pixel in each image, the distribution of intensities in a surrounding window were sampled to create a histogram and the intensities at the 50th and 99th percentiles ( and ) were calculated. A linear mapping of intensities was performed, with mapped to zero and mapped to maximum intensity. This technique counteracts the effect of systematic intensity variations through the depth of the stack. (ii) Vesselness filtering. A novel 3D vesselness filter was developed. A set of 28 directions in space was defined, giving approximately uniform coverage of a hemisphere. For each direction, a test function of position was defined as , where is the distance from the origin parallel to direction , and is the distance perpendicular to direction , and and . For a given voxel, this function was convoluted with the image stack for each of the 28 directions, and the maximum result of these was used to construct the processed image at that voxel. This test function has an elongated prolate ellipsoidal distribution, and the procedure preferentially enhances narrow filamentous structures. Because of the small size of the test function, larger structures in the image are not significantly affected by this filter. Larger vessels generally show continuous intensity in the axial direction and do not need enhancement by vesselness filtering. (iii) Fill-in filtering. Some larger vessels show low intensity in their interior, with intensely labeled walls. To fill in such structures, the following method is used. From a given voxel, a set of six lines of 25 voxels extending in each (positive and negative) coordinate direction is defined, and the maximal intensity on each line is identified. If the mean of the six maxima exceeds the intensity at the given voxel, the intensity of the given voxel is increased, by an amount that decreases exponentially with the coefficient of variation of the six maxima. This method ensures that a voxel that is surrounded in multiple directions by voxels of higher intensity receives a maximal boost in intensity. (iv) Segmentation. Using Amira, the stack was thresholded to obtain a 3D solid representing the network. (v) Skeletonization. Using Amira, the solid was skeletonized, to yield a graph consisting of nodes and edges with defined diameters. (vi) Refinement. The skeleton was further processed to combine short, connected edges and to remove tight loops, which occur as artifacts of the skeletonization. The result is a representation of the network as a set of nodes and edges, with several edges forming a segment between vessel branching points. Segment diameters were estimated as the median of edge diameters in the segment. In this study, the graphs that represent the 2PM angiograms of three animal subjects were able to enclose in a connected network more than 99.9%, 99.3%, and 99.5% of the total vessel segments, respectively. A version of the custom software used is available online ( https://github.com/secomb/StackEnhanceV1). The vessel type (e.g., arteriole, venule, or capillary) was associated with each segment by a combination of manual and automated vessel labeling.48,49 First, all pial arterioles and venules, as well as the initial segments of diving arterioles and ascending venules close to the pial surface were identified manually based on their morphology and flow direction in their branches that dive in and surface out of the cortex (e.g., penetrating arterioles and surfacing venules, respectively). Then the labeling of the remaining network was performed automatically starting from the labeled pial vascular segments and propagating the same label (e.g., arteriole or venule) down the vascular tree, excluding the microvascular segments that branch to a transverse plane. The remaining microvascular segments were labeled as capillaries. Finally, a color-coded mask of vessel types was generated based on the automatic labeling and overlaid on the angiogram stack for manual inspection and correction [Fig. 3(a)]. Fig. 3Angiogram segmentation and graphing results. (a) Overlay of vessel types on angiogram. Overlay of vessel branching order on angiogram from the (b) arteriolar side and (c) venular side. ![]() The capillary branching order starting from precapillary arterioles was computed by first assigning branching order zero to all pial and diving arterioles and subsequently assigning branching orders to the rest of the graph network starting from zero-order segments [Fig. 3(b)].49 The same procedure was then repeated for assigning the capillary branching order starting from the postcapillary venules [Fig. 3(c)]. 2.5.Coregistration of the 2PM and OCT AngiogramsAngiogram coregistration was performed by transforming the volumetric intensity images obtained by 2PM to the DLS-OCT space. To account for the non-linearity of image spaces caused by distortions, such as field curvature across those two imaging modalities due to the implementation of different optics, an initial global 3D coregistration was combined with multiple regional 2D coregistrations. The global 3D coregistration was implemented over the whole 3D 2PM and OCT stacks with an affine transformation matrix computed based on manually selected fiducial marker voxels representing the same locations in two stacks. Since 2PM and OCT image distortions change differently along the axial direction, they are difficult to address using global coregistration only. Therefore, coarse coregistration was followed by dividing the stacks along the depth dimension () into a set of -thick sub-stacks and conducting 2D coregistration of each pair of sub-stacks. The transformation matrices for 2D local coregistrations were computed from additional fiducial marker voxels manually selected in the sub-stacks after global coregistration. The results of angiogram coregistration for two example sub-stacks selected at different depths are shown in Figs. 4(a)–4(c) and Figs. 4(e)–4(g). Clear improvements are observed from applying combined two-step coregistration compared to using global 3D coregistration only [see Figs. 4(d) and 4(h)]. Herein, we applied a quick and intuitive coregistration method that can better account for the non-linear optical distortions of those two imaging modalities than the methods mainly based on affine coregistration. We also conducted a brief comparison of our method with other coregistration methods, such as the ones based on FreeSurfer,50 NiftyReg,51,52 and ANTs53,54 packages. Our method has shown improved coregistration results compared to the MRI_Robust_Register Function from the FreeSurfer package, which is mainly based on affine coregistration and comparable results to the state-of-the-art methods based on NiftyReg and ANTs, which both utilize non-linear deformable coregistration in addition to the affine coregistration (see the Supplementary Material for detailed results of the comparisons). In addition, our method enables a considerable degree of manual, user control over the coregistration process, which may be helpful to deal with sometimes significant deficiencies in acquired datasets, such as large shadows below the vessels or missing data in large sub-ROIs. Fig. 4Angiogram coregistration results. Transformed 2PM pial stack (a) (depth range: 41 to ) in DLS-OCT space and corresponding coregistration results with DLS-OCT angiogram layers (gray) overlaid by the 2PM angiogram (green) using (b) global 3D coregistration only and (c) combined global 3D coregistration and 2D regional coregistration. (d) Zoomed views of two sub-areas in stack (a) that highlights the improvements with combined 3D and 2D coregistration. Transformed 2PM in-depth stack (e) (depth range: 157 to ) in DLS-OCT space and corresponding coregistration results with (f) global 3D coregistration only and (g) combined global 3D coregistration and 2D regional coregistration. (h) Zoomed views of two sub-areas in stack (e) that highlights the improvements with combined 3D and 2D coregistration. White arrows highlight the improvements by the regional 2D coregistration. All scale bars are in length. ![]() 2.6.Blood Flow Velocity MappingBased on transformation matrices, all nodes from the graph-based network obtained from the 2PM angiogram were co-registered with the DLS-OCT volumes at different depths [Figs. 5(a) and 5(c)]. This enabled mapping of the blood flow velocities obtained by the DLS-OCT to the microvascular segments obtained by the 2PM angiography [Figs. 5(b) and 5(d)]. To assign the blood flow velocities to the microvascular segments represented by the vascular graph, a small cylindrical volume was defined in DLS-OCT space along each graph edge according to the diameter of the corresponding microvascular segment. A mean value of Vz component of the blood flow speed was subsequently extracted from each cylinder. A custom-written software was written in MATLAB (MathWorks, Massachusetts, United States) to enable manual adjustments of cylinder position, orientation, and diameter to correct for the remaining imperfections of coregistration and better match with the DLS-OCT signal from the vessel. In the case of larger vessels ( diameter) that exhibit parabolic-like flow profile, we extracted the Vz component of the maximum axial velocity in the vessel. The maximum axial velocity in larger vessels typically corresponds to the central region of the vessel. Therefore, the region from which velocities were extracted in such vessels was constrained to an -diameter cylinder around the center of the vessel. The absolute RBC flow velocities in such vessels were computed using the extracted flow velocities from the cylinder and cosine of the axial tilt angle between the corresponding graph edges and the optical axis and defined as mean center-line flow velocities. In the case of capillaries and small arterioles and venules, the extraction region was adjusted to encapsulate most of the vessel cross section since the difference between the RBC velocities from the vessel axis and periphery, if any, could not be resolved in our measurements. Absolute RBC velocities along such vessels were computed similarly using the above method, and the mean center-line flow velocities and mean flow velocities are equivalent for such vessels. Fig. 52PM vascular network graphing and coregistration with the DLS-OCT blood flow velocity measurements. The transformed graph of (a) pial and (c) in-depth 2PM stacks superimposed with top MIPs from the corresponding angiogram layers. Co-registered vascular graph and corresponding DLS-OCT axial flow velocity measurements for (b) pial and (d) in-depth stacks. All scale bars are in length. ![]() DLS-OCT measurements of the Vz component of the RBC velocity exhibit the largest and the smallest SNR when RBCs are travelling parallel and perpendicular to the optical axis (), respectively. Consequently, in the capillary network, Vz velocities were typically too noisy when vessel center-line formed a large axial tilt angle (approximately larger than 50 deg, see the Supplementary Material for details) and led to a pronounced decrease in the goodness of DLS-OCT flow velocity fitting. Therefore, we chose 35 deg as a conservative threshold for the axial tilt angle of capillary edges in data analysis to maintain a high-accuracy standard in DLS-OCT measurements while still enabling the measurements of velocities in a large number of capillary segments. Only the vascular edges with strong DLS-OCT signals were included in the data processing. However, vascular segments typically contain tens of graph edges and mean segment velocities could be obtained even if some edges within these segments needed to be removed from analysis. Examples of mapping the blood flow velocities onto a graph-based network are shown in Fig. 6. Fig. 6Visualization of axial blood flow velocity (Vz) mapping. The top and side projections of a graph-based vascular segment and its corresponding flow velocity distribution from (a) an artery, (b) a capillary, and (c) a vein. Green lines indicate edges along the segment with a white line segment highlighting the current selected edge. The white shadow areas show projections of the blood flow velocity extraction region after manual corrections. All scale bars are in length. ![]() Although most data analysis steps have been performed automatically by the custom-written software, a certain amount of manual corrections and adjustments are still needed in the graphing and coregistration procedures for refined blood flow speed mapping, which is time consuming. In the future, machine learning-based methods may be developed to assist with the manual-correction steps. 3.Results of In Vivo Measurements and DiscussionThe mean center-line flow velocity distributions in cortical arterioles, capillaries, and venules based on measurements in three mice are shown in Fig. 7(a) and summarized in Table 1. The total numbers of graph edges and segments whose flow velocities were accounted for are 1554 edges/454 segments, 925 edges/316 segments, and 1613 edges/549 segments for mouse #1, #2, and #3, respectively. Herein, using the proposed method, we were able to combine microvascular flow velocities with vascular morphological information on a significantly larger scale than previously reported.55 Compared to arterioles, capillaries and venules exhibited 82.1% and 57.1% lower average center-line flow velocities. This is in agreement with previously published measurements55 and expected in the mouse cortex as there is larger collective cross-sectional area of venules than arterioles, and even larger collective cross-sectional area of capillaries than venules. Fig. 7Quantitative analysis of the microvascular velocities obtained from 3 mice. (a) Mean center-line flow velocity distribution in arterioles, capillaries, and venules as labeled with the proposed strategy (454, 316, and 549 vessel segments from mouse #1, #2, and #3, respectively, were used for calculation). Mean flow velocity distributions in capillaries of the first four branching orders from (b) precapillary arterioles and (c) postcapillary venules. ![]() Table 1Mean center-line blood flow velocities in arterioles, capillaries, and venules.
The mean flow velocity distributions in capillaries of the first four branching orders from precapillary arterioles and postcapillary venules are shown in Figs. 7(b) and 7(c) and summarized in Table 2, respectively. Due to truncation of the microvascular networks at the boundaries of the imaged volumes, the assignment of the capillary branching order was reliable only for the first 3 to 4 branching orders from either precapillary arterioles or postcapillary venules. A decreasing trend of capillary flow velocity was observed at the first four branching orders following precapillary arterioles (from 4.3 to ), whereas mean flow velocity was similar across the capillaries of the first four branching orders counted from the postcapillary venules (). A similar trend was previously observed in mice, where average RBC speed in capillaries decreased from to for the first four branching orders following precapillary arterioles while it remained for capillaries in the vicinity of postcapillary venules.55 This trend of rapid capillary flow speed decrease within the first several branching orders following the feeding penetrating arteriole can be explained by successive vessel bifurcation leading to fast increase of collective cross-sectional area. However, this trend gradually fades away toward the postcapillary venules due to a highly interconnected capillary bed.56 Table 2Mean flow velocities in capillaries of the first four branching orders from precapillary arterioles and postcapillary venules.
4.ConclusionWe have proposed a method to combine the high-resolution cerebral microvascular angiograms obtained by 2PM and large-scale microvascular blood flow velocity measurements based on DLS-OCT for a comprehensive analysis of cerebral microvascular blood flow network down to capillary level in mice. The developed methodology was applied in proof-of-principle experiments to retrieve the distributions of mean blood flow velocities from several hundred arterioles, capillaries, and venules in vivo. We anticipate that this technique will be helpful for quantifying the microvascular blood flow velocity distributions in a broad range of studies involving normal brain functioning, progression of various microvascular diseases, and numerical modeling of the oxygen advection and diffusion in the realistic microvascular networks.57 Code, Data, and Materials AvailabilityData and codes used in the work are available online ( https://github.com/qipianmgh/MicroscopicFlowMapping) or upon request. AcknowledgmentsThis work was supported by the National Institutes of Health (Grant Nos. R01NS115401, U24EB028941, U01HL133362, and R01NS091230). ReferencesA. A. Phillips et al.,
“Neurovascular coupling in humans: physiology, methodological advances and clinical implications,”
J. Cereb. Blood Flow Metab., 36
(4), 647
–664 https://doi.org/10.1177/0271678X15617954
(2016).
Google Scholar
L. Kaplan, B. W. Chow and C. Gu,
“Neuronal regulation of the blood–brain barrier and neurovascular coupling,”
Nat. Rev. Neurosci., 21
(8), 416
–432 https://doi.org/10.1038/s41583-020-0322-2 NRNAAN 1471-003X
(2020).
Google Scholar
F. A. Sorond et al.,
“Neurovascular coupling, cerebral white matter integrity, and response to cocoa in older people,”
Neurology, 81
(10), 904
–909 https://doi.org/10.1212/WNL.0b013e3182a351aa NEURAI 0028-3878
(2013).
Google Scholar
S. Ogoh,
“Relationship between cognitive function and regulation of cerebral blood flow,”
J. Physiol. Sci., 67
(3), 345
–351 https://doi.org/10.1007/s12576-017-0525-0
(2017).
Google Scholar
M. Sforza et al.,
“The impact of cerebral vasomotor reactivity on cerebrovascular diseases and cognitive impairment,”
J. Neural Transm., 129
(11), 1321
–1330 https://doi.org/10.1007/s00702-022-02546-w
(2022).
Google Scholar
N. K. Logothetis et al.,
“Neurophysiological investigation of the basis of the fMRI signal,”
Nature, 412
(6843), 150
–157 https://doi.org/10.1038/35084005
(2001).
Google Scholar
H. F. Wehrl et al.,
“Simultaneous PET-MRI reveals brain function in activated and resting state on metabolic, hemodynamic and multiple temporal scales,”
Nat. Med., 19
(9), 1184
–1189 https://doi.org/10.1038/nm.3290 1078-8956
(2013).
Google Scholar
C. Iadecola,
“The neurovascular unit coming of age: a journey through neurovascular coupling in health and disease,”
Neuron, 96
(1), 17
–42 https://doi.org/10.1016/j.neuron.2017.07.030 NERNET 0896-6273
(2017).
Google Scholar
T. L. Stackhouse and A. Mishra,
“Neurovascular coupling in development and disease: focus on astrocytes,”
Front. Cell Dev. Biol., 9 702832 https://doi.org/10.3389/fcell.2021.702832
(2021).
Google Scholar
S. Tarantini et al.,
“Impaired neurovascular coupling in aging and Alzheimer’s disease: contribution of astrocyte dysfunction and endothelial impairment to cognitive decline,”
Exp. Gerontol., 94 52
–58 https://doi.org/10.1016/j.exger.2016.11.004 EXGEAB 0531-5565
(2017).
Google Scholar
K. Kotliar et al.,
“Altered neurovascular coupling as measured by optical imaging: a biomarker for Alzheimer’s disease,”
Sci. Rep., 7
(1), 12906 https://doi.org/10.1038/s41598-017-13349-5 SRCEC3 2045-2322
(2017).
Google Scholar
L. Park et al.,
“tPA deficiency underlies neurovascular coupling dysfunction by amyloid-β,”
J. Neurosci., 40
(42), 8160
–8173 https://doi.org/10.1523/JNEUROSCI.1140-20.2020 JNRSDS 0270-6474
(2020).
Google Scholar
L. Li et al.,
“Impaired hippocampal neurovascular coupling in a mouse model of Alzheimer’s disease,”
Front. Physiol., 12 715446 https://doi.org/10.3389/fphys.2021.715446 FROPBK 0301-536X
(2021).
Google Scholar
J. L. Presa et al.,
“Vasculo-neuronal coupling and neurovascular coupling at the neurovascular unit: impact of hypertension,”
Front. Physiol., 11 584135 https://doi.org/10.3389/fphys.2020.584135 FROPBK 0301-536X
(2020).
Google Scholar
O. Shabir et al.,
“Assessment of neurovascular coupling and cortical spreading depression in mixed mouse models of atherosclerosis and Alzheimer’s disease,”
Elife, 11 e68242 https://doi.org/10.7554/eLife.68242
(2022).
Google Scholar
O. Prager et al.,
“Seizure‐induced microvascular injury is associated with impaired neurovascular coupling and blood–brain barrier dysfunction,”
Epilepsia, 60
(2), 322
–336 https://doi.org/10.1111/epi.14631 EPILAK 0013-9580
(2019).
Google Scholar
L. Gagnon et al.,
“Modeling of cerebral oxygen transport based on in vivo microscopic imaging of microvascular network structure, blood flow, and oxygenation,”
Front. Comput. Neurosci., 10 82 https://doi.org/10.3389/fncom.2016.00082 1662-5188
(2016).
Google Scholar
L. Gagnon et al.,
“Multimodal reconstruction of microvascular-flow distributions using combined two-photon microscopy and Doppler optical coherence tomography,”
Neurophotonics, 2
(1), 015008 https://doi.org/10.1117/1.NPh.2.1.015008
(2015).
Google Scholar
A. Y. Shih et al.,
“Two-photon microscopy as a tool to study blood flow and neurovascular coupling in the rodent brain,”
J. Cereb. Blood Flow Metab., 32
(7), 1277
–1309 https://doi.org/10.1038/jcbfm.2011.196
(2012).
Google Scholar
J.-Y. Huang et al.,
“In vivo two-photon fluorescence microscopy reveals disturbed cerebral capillary blood flow and increased susceptibility to ischemic insults in diabetic mice,”
CNS Neurosci. Ther., 20
(9), 816
–822 https://doi.org/10.1111/cns.12268
(2014).
Google Scholar
B. Li et al.,
“Two-photon microscopic imaging of capillary red blood cell flux in mouse brain reveals vulnerability of cerebral white matter to hypoperfusion,”
J. Cereb. Blood Flow Metab., 40
(3), 501
–512 https://doi.org/10.1177/0271678X19831016
(2020).
Google Scholar
V. J. Srinivasan et al.,
“Quantitative cerebral blood flow with optical coherence tomography,”
Opt. Express, 18
(3), 2477
–2494 https://doi.org/10.1364/OE.18.002477 OPEXFF 1094-4087
(2010).
Google Scholar
M. A. Yaseen et al.,
“Microvascular oxygen tension and flow measurements in rodent cerebral cortex during baseline conditions and functional activation,”
J. Cereb. Blood Flow Metab., 31
(4), 1051
–1063 https://doi.org/10.1038/jcbfm.2010.227
(2011).
Google Scholar
J. Lee et al.,
“Multiple-capillary measurement of RBC speed, flux, and density with optical coherence tomography,”
J. Cereb. Blood Flow Metab., 33
(11), 1707
–1710 https://doi.org/10.1038/jcbfm.2013.158
(2013).
Google Scholar
L. Qi et al.,
“Fully distributed absolute blood flow velocity measurement for middle cerebral arteries using Doppler optical coherence tomography,”
Biomed. Opt. Express, 7
(2), 601
–615 https://doi.org/10.1364/BOE.7.000601 BOEICL 2156-7085
(2016).
Google Scholar
J. Yao et al.,
“High-speed label-free functional photoacoustic microscopy of mouse brain in action,”
Nat. Methods, 12
(5), 407
–410 https://doi.org/10.1038/nmeth.3336 1548-7091
(2015).
Google Scholar
B. Ning et al.,
“Simultaneous photoacoustic microscopy of microvascular anatomy, oxygen saturation, and blood flow,”
Opt. Lett., 40
(6), 910
–913 https://doi.org/10.1364/OL.40.000910 OPLEDP 0146-9592
(2015).
Google Scholar
F. Zhong et al.,
“High-speed wide-field multi-parametric photoacoustic microscopy,”
Opt. Lett., 45
(10), 2756
–2759 https://doi.org/10.1364/OL.391824 OPLEDP 0146-9592
(2020).
Google Scholar
R. Cao et al.,
“Hemodynamic and oxygen-metabolic responses of the awake mouse brain to hypercapnia revealed by multi-parametric photoacoustic microscopy,”
J. Cereb. Blood Flow Metab., 41
(10), 2628
–2639 https://doi.org/10.1177/0271678X211010352
(2021).
Google Scholar
C. Errico et al.,
“Ultrafast ultrasound localization microscopy for deep super-resolution vascular imaging,”
Nature, 527
(7579), 499
–502 https://doi.org/10.1038/nature16066
(2015).
Google Scholar
K. Christensen-Jeffries et al.,
“In vivo acoustic super-resolution and super-resolved velocity mapping using microbubbles,”
IEEE Trans. Med. Imaging, 34
(2), 433
–440 https://doi.org/10.1109/TMI.2014.2359650 ITMID4 0278-0062
(2015).
Google Scholar
K. Christensen-Jeffries et al.,
“Super-resolution ultrasound imaging,”
Ultrasound Med. Biol., 46
(4), 865
–891 https://doi.org/10.1016/j.ultrasmedbio.2019.11.013 USMBA3 0301-5629
(2020).
Google Scholar
O. Demeulenaere et al.,
“In vivo whole brain microvascular imaging in mice using transcranial 3D ultrasound localization microscopy,”
eBioMedicine, 79 103995 https://doi.org/10.1016/j.ebiom.2022.103995
(2022).
Google Scholar
R. Lu et al.,
“Rapid mesoscale volumetric imaging of neural activity with synaptic resolution,”
Nat. Methods, 17
(3), 291
–294 https://doi.org/10.1038/s41592-020-0760-9 1548-7091
(2020).
Google Scholar
J. T. Giblin et al.,
“Measuring capillary flow dynamics using interlaced two-photon volumetric scanning,”
J. Cereb. Blood Flow Metab., 43
(4), 595
–609 https://doi.org/10.1177/0271678X221145091
(2023).
Google Scholar
G. Meng et al.,
“Ultrafast two-photon fluorescence imaging of cerebral blood circulation in the mouse brain in vivo,”
Proc. Natl. Acad. Sci. U. S. A., 119
(23), e2117346119 https://doi.org/10.1073/pnas.2117346119
(2022).
Google Scholar
J. Yao and L. V. Wang,
“Photoacoustic microscopy,”
Laser Photonics Rev., 7
(5), 758
–778 https://doi.org/10.1002/lpor.201200060
(2013).
Google Scholar
V. J. Srinivasan et al.,
“OCT methods for capillary velocimetry,”
Biomed. Opt. Express, 3
(3), 612
–629 https://doi.org/10.1364/BOE.3.000612 BOEICL 2156-7085
(2012).
Google Scholar
W. J. Choi et al.,
“Cerebral capillary velocimetry based on temporal OCT speckle contrast,”
Biomed. Opt. Express, 7
(12), 4859
–4873 https://doi.org/10.1364/BOE.7.004859 BOEICL 2156-7085
(2016).
Google Scholar
J. Lee et al.,
“Dynamic light scattering optical coherence tomography,”
Opt. Express, 20
(20), 22262
–22277 https://doi.org/10.1364/OE.20.022262 OPEXFF 1094-4087
(2012).
Google Scholar
J. Lee et al.,
“Quantitative imaging of cerebral blood flow velocity and intracellular motility using dynamic light scattering-optical coherence tomography,”
J. Cereb. Blood Flow Metab., 33
(6), 819
–825 https://doi.org/10.1038/jcbfm.2013.20
(2013).
Google Scholar
J. Tang et al.,
“Shear‐induced diffusion of red blood cells measured with dynamic light scattering‐optical coherence tomography,”
J. Biophotonics, 11
(2), e201700070 https://doi.org/10.1002/jbio.201700070
(2018).
Google Scholar
Y. Zhao et al.,
“Phase-resolved optical coherence tomography and optical Doppler tomography for imaging blood flow in human skin with fast scanning speed and high velocity sensitivity,”
Opt. Lett., 25
(2), 114
–116 https://doi.org/10.1364/OL.25.000114 OPLEDP 0146-9592
(2000).
Google Scholar
S. Stefan and J. Lee,
“Deep learning toolbox for automated enhancement, segmentation, and graphing of cortical optical coherence tomography microangiograms,”
Biomed. Opt. Express, 11
(12), 7325
–7342 https://doi.org/10.1364/BOE.405763 BOEICL 2156-7085
(2020).
Google Scholar
M. A. Yaseen et al.,
“Multimodal optical imaging system for in vivo investigation of cerebral oxygen delivery and energy metabolism,”
Biomed. Opt. Express, 6
(12), 4994
–5007 https://doi.org/10.1364/BOE.6.004994 BOEICL 2156-7085
(2015).
Google Scholar
S. Almasi et al.,
“A novel method for identifying a graph-based representation of 3D microvascular networks from fluorescence microscopy image stacks,”
Med. Image Anal., 20
(1), 208
–223 https://doi.org/10.1016/j.media.2014.11.007
(2015).
Google Scholar
R. Damseh et al.,
“Automatic graph-based modeling of brain microvessels captured with two-photon microscopy,”
IEEE J. Biomed. Heal. Inf., 23
(6), 2551
–2562 https://doi.org/10.1109/JBHI.2018.2884678
(2019).
Google Scholar
S. Sakadžić et al.,
“Two-photon high-resolution measurement of partial pressure of oxygen in cerebral vasculature and tissue,”
Nat. Methods, 7
(9), 755
–759 https://doi.org/10.1038/nmeth.1490 1548-7091
(2010).
Google Scholar
S. Sakadžić et al.,
“Large arteriolar component of oxygen delivery implies a safe margin of oxygen supply to cerebral tissue,”
Nat. Commun., 5
(1), 5734 https://doi.org/10.1038/ncomms6734 NCAOBW 2041-1723
(2014).
Google Scholar
M. Reuter, H. D. Rosas and B. Fischl,
“Highly accurate inverse consistent registration: a robust approach,”
Neuroimage, 53
(4), 1181
–1196 https://doi.org/10.1016/j.neuroimage.2010.07.020 NEIMEF 1053-8119
(2010).
Google Scholar
M. Modat et al.,
“Fast free-form deformation using graphics processing units,”
Comput. Methods Programs Biomed., 98
(3), 278
–284 https://doi.org/10.1016/j.cmpb.2009.09.002 CMPBEK 0169-2607
(2010).
Google Scholar
M. Modat et al.,
“Global image registration using a symmetric block-matching approach,”
J. Med. Imaging, 1
(2), 024003 https://doi.org/10.1117/1.JMI.1.2.024003 JMEIET 0920-5497
(2014).
Google Scholar
B. Avants et al.,
“Symmetric diffeomorphic image registration with cross-correlation: evaluating automated labeling of elderly and neurodegenerative brain,”
Med. Image Anal., 12
(1), 26
–41 https://doi.org/10.1016/j.media.2007.06.004
(2008).
Google Scholar
B. B. Avants et al.,
“A unified image registration framework for ITK,”
in Proceedings of the 5th Workshop on Biomedical Image Registration LNCS 7359,
266
–275
(2012). Google Scholar
T. P. Santisakultarm et al.,
“In vivo two-photon excited fluorescence microscopy reveals cardiac- and respiration-dependent pulsatile blood flow in cortical blood vessels in mice,”
Am. J. Physiol. Circ. Physiol., 302
(7), H1367
–H1377 https://doi.org/10.1152/ajpheart.00417.2011
(2012).
Google Scholar
I. G. Gould et al.,
“The capillary bed offers the largest hemodynamic resistance to the cortical blood supply,”
J. Cereb. Blood Flow Metab., 37
(1), 52
–68 https://doi.org/10.1177/0271678X16671146
(2017).
Google Scholar
J. T. Celaya-Alcala et al.,
“Simulation of oxygen transport and estimation of tissue perfusion in extensive microvascular networks: application to cerebral cortex,”
J. Cereb. Blood Flow Metab., 41
(3), 656
–669 https://doi.org/10.1177/0271678X20927100
(2021).
Google Scholar
|
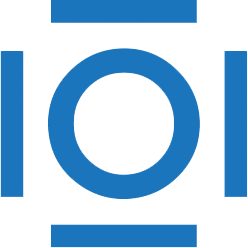
CITATIONS
Cited by 1 scholarly publication.
Angiography
Blood circulation
Capillaries
Optical coherence tomography
Biological imaging
Brain
Image segmentation